AI Alignment Through Anthropology: How Social Science Can Steer AI Towards Better Outcomes
Aligning AI developers with human values through anthropology. Originally published for the Anthropology + Technology Conference 2021.
ANTHROPOLOGYSOCIAL SCIENCEARTIFICIAL INTELLIGENCEANI
3/19/20214 min read
If an advanced AI system were instructed to make paper clips, or to fetch coffee, we would not want it to carry out this task at any cost. For example, we would rather the AI not kill anyone in the process or use valuable resources that ought to be used for other purposes. Rather, we want the AI to know how to achieve this goal in a way that’s consistent with human values (i.e. valuing a human life over coffee or valuable resources more than paper clips). Figuring out how to design AI systems so that they do not inadvertently act in ways that would be contrary to human values is known as the Value Alignment Problem.
Typically, approaches to the Value Alignment Problem involve two assumptions:
The problem concerns future AGI (Artificial General Intelligence) rather than present day ANI (Artificial Narrow Intelligence).
The aim is to align the AI with human values rather than align the AI designers.
It’s no revelation to point out misalignment between ANI and humans today, nor that AI designers need to better understand their users’ values. Instead, we’re proposing that an anthropological perspective can help reframe the Value Alignment Problem (VAP).
Why We Should Focus on ANI
It is evident from how often we hear of AI ‘fails’ that misalignment between AI and stakeholders is a significant and recurring problem. Yet the assumption that the VAP concerns future AGI ignores problems evident in ANI today. This is troubling for a number of reasons. Firstly, failing to consider current harms blinkers developers from looking for solutions in the world around them. Second and more importantly, there can be damaging outcomes for the end-users of AI when their real world contexts are not fully considered.
Take Microsoft’s failed launch of Tay the chatbot in 2016. Tay was built to ‘learn’ from Twitter users and develop casual conversational skills. Yet within 24 hours had been corrupted by bad actors on the platform who trained the AI to spout racist and offensive statements. Though trolls are an undesirable part of Twitter’s user base, overlooking them was both naïve and indicative of a significant blindspot in the developers’ view of the Twitter landscape.
In other instances, assuming the VAP is a problem for AGI in the future, not the AI of the present can be fatal. This is evidenced by the case of 14-year old Molly Russell who committed suicide after going down a “dark rabbit hole of depressive suicidal content” on Instagram. When recommendation algorithms use past behaviour to predict what kind of content you would like to see more of, it can trap users in vortexes of similar content. In this case, and many others, this vortex can be extreme, harmful and for those trying to escape it, difficult to climb out of. The harmful outcomes of these AIs expose how designers have neglected to consider or show significant empathy for their contexts of use. “Operating in a bubble and ignoring the current needs of society” is, as Francesca Gadeleta, Chief Data Officer at Abe.ai, states “a sure path to failure”.
How Anthropology Can Help to Tackle the Value Alignment Problem
Secondly, conventional approaches to the VAP assume that the solution lies in AI being better aligned with human values. Whilst important, this emphasis takes the onus away from the people who are directly responsible for AI: the designers and developers themselves. Without aligning AI developers with the values of those who use and are affected by their products, misalignment between people and AI will be a persistent problem.
Had Tay’s creators sufficiently considered how people behave in communication with chatbots, and with other humans on large social media platforms — in particular the desire to corrupt and break things — it may have been designed with better guardrails in place. And if Instagram had a greater awareness of viewing desires and habits, it might have changed the algorithm to prevent these “dark rabbit holes” from emerging. This second example highlights the cost of relying purely on usage metrics, instead of nuanced human insight. In the absence of some (probably mythical) AGI of the future that could sufficiently understand human intent or values, responsibility falls to AI designers today to better understand the context their technology is used in and how people interact with it.
Increasingly there are calls for a more interdisciplinary approach to AI development. Organisations such as OpenAI, AI Now, and the Ada Lovelace Institute realise the value of drawing on social science perspectives. Randy Connelly has outlined some practical solutions for addressing this at university level, such as diversifying faculty hires and having more social science modules included in computing teaching. Yet there is more immediate work to be done: engaging AI designers and developers directly with disciplines like anthropology, and engaging anthropology with those working in technology.
Anthropological perspectives and methodologies can offer developers significant contextual understanding of stakeholders’ worlds and how they are affected by their technology. Often this involves drawing out the nuances in values and behaviours that sit outside a development team’s direct personal experience. Much of this can’t be captured by metrics, and demands a human centred approach to uncover the variety of values held across and within different cultures.
Integrating anthropological thinking and methods within AI design and development is one part of the solution to better alignment with stakeholder values. Ultimately, having a broad range of culturally diverse people working in AI is essential so that the broadest set of assumptions and implicit understandings of how the world works are brought to bear on what’s created. Without diverse perspectives from inside, there is a risk of creating AIs that are blind to the context they operate in. At worst, this risks marginalising the underrepresented, as argued by Abeba Birhane:
“People create, control, and are responsible for any system. For the most part such people consist of a homogeneous group of predominantly white, middle-class males from the Global North. Like any other tool, AI is one that reflects human inconsistencies, limitations, biases, and the political and emotional desires of the individuals behind it and the social and cultural ecology that embed it. Just like a mirror that reflects how society operates – unjust and prejudiced against some individuals and communities.”
Conclusion
The VAP will eventually be solved once those developing AI themselves have a better understanding of stakeholder values and design their technologies accordingly. To echo Jonathan Stray, this work also needs to happen now in order to reap the benefits down the line: “narrow alignment is worth working on for its own sake, and may also give us critical insight into the general alignment problem.” Equipped with a better understanding of user context, designers and developers will be better situated to minimise harms and maximise AI’s utility. Learning from anthropologists is one piece of that puzzle.
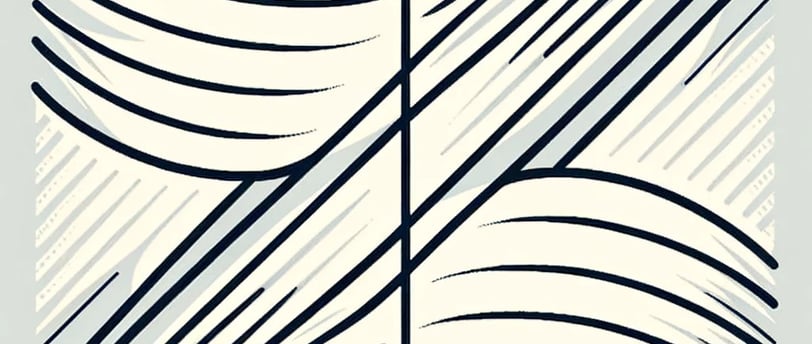
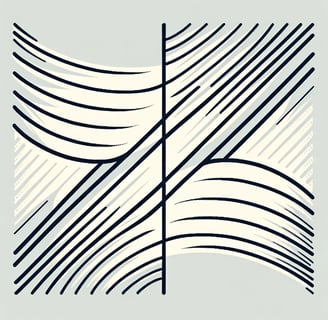